You have /5 articles left.
Sign up for a free account or log in.
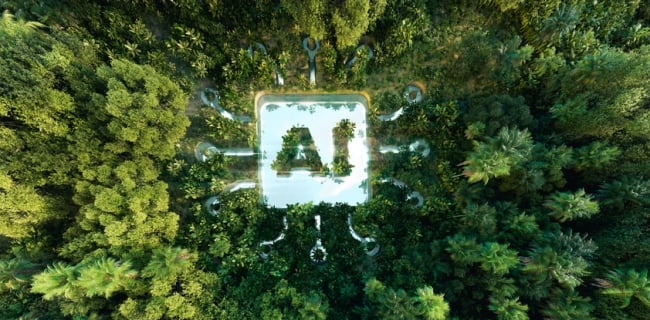
Petmal/iStock/Getty Images Plus
College teaching has always required the consumption of natural resources, but with the rapid adoption of generative artificial intelligence tools, it is poised to become much more resource-intensive.
You may have seen the steady stream of news headlines about the significant energy needs of artificial intelligence, including “AI Needs So Much Power That Old Coal Plants Are Sticking Around,” “Amid Explosive Demand, America Is Running Out of Power” or “AI Is Exhausting the Power Grid. Tech Firms Are Seeking a Miracle Solution.” What is going on?
The answer comes down to one word: “compute.” If the term is new to you, the AI Now Institute, a policy research institute focused on the social effects of AI, offers a helpful primer on computational power and AI. Briefly, compute, short for computational power, can refer to the amount of hardware (semiconductor chips), software (the computations running on those chips) or both used to complete a computational task. The large language models—LLMs—that power tools like Google’s Gemini, Microsoft’s Copilot and OpenAI’s ChatGPT are computationally intensive to create and run. The computational intensity of LLMs means that their potential environmental effects are greater than those of the technologies most faculty and students are accustomed to using in the classroom.
Many experts in higher ed are debating important questions about the implications of LLMs for student learning. In my own teaching of academic writing, I spent significant time last year exploring what knowledge, skills and habits of mind might be enhanced—or undermined—by allowing or encouraging students to use tools like ChatGPT. The answers to these questions are far from settled for me, but as I think ahead to teaching in the next academic year, I am considering an additional set of questions: How many kilowatt hours of electricity am I willing to spend to support a class meeting in which my students research and brainstorm ideas for a new essay? How many gallons of potable water would I be willing to pour down a drain to reach a course’s learning goals? How many ounces of conflict minerals would I want to be mined to support my students’ composition of essays next term and across the years ahead?
In discussions of the effects of generative AI on college teaching, questions about sustainability are often overlooked or sometimes noted in passing and then ignored. These questions deserve our focused attention. In order to understand why they matter, college faculty must understand the concerns being raised about the environmental effects of LLMs.
A recent multimodal news feature in Bloomberg, “AI Is Already Wreaking Havoc on Global Power Systems,” begins with an aerial image of a patch of mostly undeveloped land in Loudoun County in Northern Virginia, “once known for its horse farms and Civil War battle sites.” As the reader scrolls, the land evolves between 2002 and 2024, filling up with data centers. As the article explains, powering the creation and running of LLMs is straining our electrical grids. The number of data centers built or being built around the world has gone from 3,600 in 2015 to more than 7,000 in 2024; by 2034, these centers may require 1,580 terawatt hours of electricity per year, about as much electricity as the nation of India uses annually.
At an event on the sidelines of the World Economic Forum at Davos this year, OpenAI CEO Sam Altman said the future of AI will require an energy breakthrough. Altman has invested $375 million of his personal fortune in Helion Energy, a nuclear fusion company.
In addition to the rapidly increasing electricity needs associated with artificial intelligence, many data centers also require a lot of water. In Southern California, where I teach, renewable energy goals seem potentially achievable, but water scarcity is a more intractable problem. In an introduction to data centers and water use published in the open-access journal npj Clean Water, David Mytton explains that in data centers, water is used both indirectly, through electricity use, and directly, for cooling, which is crucial because the integrated circuits inside data centers produce heat as a by-product. If they get too hot, they stop working.
As Mytton explains, there are different methods for reducing heat in data centers, and the “general approach” involves the direct use of water. In some data centers, this water is recycled, but in many cases, it is cost-prohibitive or otherwise difficult to do so, and potable water is often used to keep equipment cool. (It is possible to build data centers that use cool air from outdoors, or even seawater, to regulate the temperature inside, but typically only in specific environments.)
Mytton calls for better transparency about water use in data centers, but even when that information exists, it is not easily accessible to an individual user of software supported by that center. According to a preprint paper by researchers at the University of California, Riverside, and the University of Texas at Arlington, 10 inferences (queries) by a GPT-3 user in the state of Washington require the consumption of 500 milliliters of water (about 2.1 cups), while a user in Ireland could make 70 inferences using the same amount of water.
LLMs depend on state-of-the-art processing chips. The manufacturing of these chips, such as those in Nvidia’s graphics processing units (GPUs), is energy- and water-intensive, in addition to requiring a range of mined metals and minerals like cobalt, gold, mica, tantalum, tin and tungsten. (Tin, tantalum, tungsten and gold, referred to as 3TG, are classified by Section 1502 of the Dodd-Frank Act as conflict minerals.) Nvidia’s Fiscal Year 2024 Sustainability Report describes the company’s efforts to vet suppliers to source conflict-free minerals and ensure a responsible supply chain, but even under the best conditions, extracting these materials from the earth is a destructive, grueling and toxic undertaking.
To their credit, Amazon, Microsoft and Google (owned by Alphabet) have all set ambitious sustainability goals. However, goals do not guarantee results. In 2020, Microsoft pledged to remove more carbon from the atmosphere than it emits by 2030. But Microsoft’s 2024 Sustainability Report, released in May, indicated that its carbon emissions have increased 29 percent since 2020: as Bloomberg reported, this was “one of the first concrete examples of how the pursuit of AI is colliding with efforts to cut emissions.” Microsoft’s total water consumption is likewise on an upward trajectory, up 87 percent since 2020.
Similarly, Google released its 2024 Environmental Report in July, disclosing that despite its goal to reach net zero carbon emissions by 2030, its emissions grew 13 percent in 2023, an increase “primarily driven by increased data center energy consumption and supply chain emissions.” Since 2019, Google’s emissions are up 48 percent. Google’s water consumption at its data centers and offices increased 14 percent from 2022 to 2023, an increase the company attributes to data center cooling needs.
Businesses must answer to shareholders and investors, and their leaders have a fiduciary responsibility to pursue profit and growth. (OpenAI’s unique corporate structure and mission could put it in a somewhat different position with regard to profits than its publicly traded competitors, though this seems increasingly unlikely.) Currently, the industry leaders’ near-term goals of market dominance in generative AI are overriding some of their sustainability goals.
Google’s chief sustainability officer, Kate Brandt, told the Associated Press in July that reaching Google’s goal of net zero emissions and carbon-free energy by 2030 will not be easy, and “will require us to navigate a lot of uncertainty, including this uncertainty around the future of AI’s environmental impacts.” We must hope that these businesses will continue to find that sustainability aligns with their business interests over the next decade, and university leaders should use any leverage they have to ensure this is the case.
The uncertainty Brandt notes is real. Researchers are only beginning to understand the current and future demands large language models will put on the environment. It remains to be seen if researchers’ suggestions for best practices take hold and whether new technological developments can reduce the environmental impact of these tools. The National Science Foundation is investing heavily in bold research projects focused on sustainable computing. But more sustainable computing practices often cost money to implement, so we cannot assume that good research in this area will resolve this issue: Businesses must decide to act in response to it.
One of the most interesting findings from recent research on the energy costs of machine learning comes from researchers at Hugging Face and Carnegie Mellon University. They found that among various machine learning models, “the most energy- and carbon-intensive tasks are those that generate new content: text generation, summarization, image captioning, and image generation.” Further, they found that using multipurpose models for specific tasks uses more energy than using a task-specific model. For example, if you ask a model specifically trained to summarize texts to summarize a text and also ask a model that has been trained to respond to many different requests to summarize a text, the latter requires more energy. If replicated across newer LLMs, these conclusions have implications for how these tools could be least harmfully used in college teaching. Hopefully, future research will make it possible for faculty across disciplines to access evidence-based practices for the sustainable use of LLMs.
For now, as faculty consider making major transformations to our teaching, we lack important information we need about the sustainability of generative AI tools. Many who are redesigning courses and curricula remain unaware of the possible environmental impacts of incorporating these tools widely into teaching and learning. My argument is not that college instructors should broadly oppose the use of generative AI in teaching because it may negatively impact the environment: Many daily activities require natural resources and produce carbon emissions, and we must constantly make difficult choices about using resources to accomplish goals we believe are important.
However, I am concerned about how easy it may be to use generative AI tools in foolishly wasteful ways without even being aware we are doing so. Faculty decisions to use tools based on LLMs should be judicious rather than playful or casual, and we should try to take into consideration the full range of effects. When we decide to use these tools in courses, our teaching must include discussions about the possible effects, including environmental impacts.
I am further concerned universities may rush to build digital infrastructures in which wasteful uses of LLMs are built in and automatic, happening without any direct effort or decision on an instructor’s or a student’s part. In a commentary exploring the energy implications of AI, Alex de Vries cautions developers to “critically consider the necessity of using AI in the first place, as it is unlikely that all applications will benefit from AI or that the benefits will always outweigh the costs.” Educational technologists must take this advice seriously.
Individual instructors need the help of university leaders to ensure we can teach in a sustainable way in the years ahead. Campus sustainability plans must factor in the costs of incorporating LLM-based tools into teaching, research and other university operations. Critical discussion of sustainability with those creating and selling these tools to universities should also be a priority. OpenAI announced ChatGPT Edu on May 30, touting it as a version of its product that will meet the high security and privacy needs of users in universities. Clearly, OpenAI sees university faculty, staff and students as possible long-term, reliable customers for its product, and no doubt its competitors do as well. However, nothing on the ChatGPT Edu press release webpage anticipates concerns among university users about sustainability. I encourage university administrators, IT professionals and faculty involved in upcoming negotiations with OpenAI and other generative AI vendors to clearly communicate that sustainability and transparency about the environmental impacts of their products are high priorities for university customers.
On Aug. 10, 2023, Sam Altman posted on X, “No one knows what happens next.” There’s no doubt the statement is true, and this can be both terrifying and exhilarating. Still, university leaders can exert their power to try to influence what happens next in our corner of the generative AI marketplace. University faculty can communicate to leadership that we care about sustainability and need information about the environmental impacts of the educational technology we have access to in order to make responsible choices about its use. Faculty can also affect the future through our ongoing work with students. We must prepare them to thrive in a world where these technologies will change their work and lives while teaching them to apprehend the issues so they can make the difficult choices required to reduce carbon emissions and steward natural resources.