You have /5 articles left.
Sign up for a free account or log in.
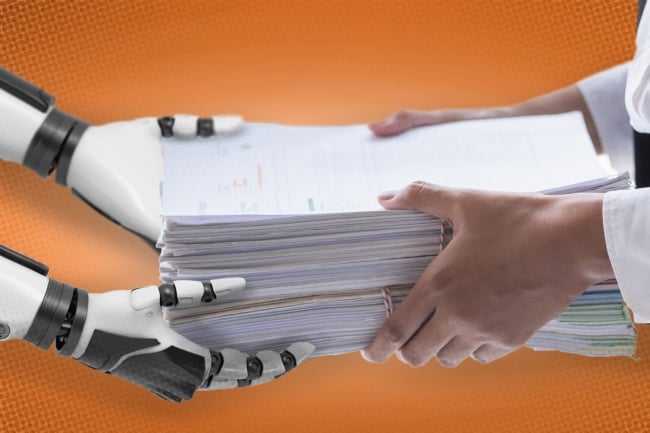
Photo illustration by Justin Morrison/Inside Higher Ed | David Gyung and Gearstd/iStock/Getty Images
Informa, the academic publishing powerhouse and parent company of Routledge and Taylor & Francis, recently announced a deal with Microsoft that will feed a massive body of scholarly work to a generative AI system. Like many academics, I was stunned by the news, which meant that my most recently published book would be used in a way that I could not have envisioned when I signed my contract. After inquiring with my editor, who informed me that Informa had entered into not one but two contracts with AI companies to provide content for training, I was told that my contract was airtight and there was no opt-out option for my book.
If Informa’s decision portends a wave of similar deals between scholarly publishers and generative AI companies, the troubling precedent this sets could result in significant changes to the nature of academic publishing. My editor was unable to provide additional information about the terms of the deal other than what were publicly released in the official announcement. With so much mystery surrounding the precise details of this seismic announcement, anyone who has or will consider publishing with one of Informa’s vast portfolio of academic outlets is left with a series of lingering questions.
To begin, Informa insists that the purpose of this deal is to “help improve relevance and performance of AI systems,” indicating their hope that the use of scholarship will make generative AI systems more reliable. The first question we must ask, therefore, is to what extent this lofty goal is possible.
Optimistically, large language models could improve simply by incorporating information that has been subject to quality control in the form of peer review, supplementing dirty data with clean. But there are three big reasons why we should be skeptical that academic content can solve AI’s problems. First, incorporating new scholarly data will not by itself solve the problem of hallucinations, where LLMs produce incorrect or made-up answers to prompted questions. Though processes like retrieval augmented generation show promise to reduce the problem, hallucinations have to date remained a stubborn feature of the technology itself, especially for the most high-profile general purpose LLMs.
Second, LLMs are truth agnostic. They use probabilistic algorithmic models to produce a likely answer to prompted questions. Whether information is accurate or false is beside the point. At their current stage of development, therefore, LLMs are unable to reliably distinguish between quality, peer-reviewed work and other information that the systems are already trained on. Which then raises the third problem, that many of the most high-profile general purpose LLMs have been trained on information scraped from the internet, and they have been known to reproduce biased content, among other potential risks posed by generative AI. Without significant structural changes, incorporating scholarly data into many existing for-profit generative AI systems will be the metaphorical equivalent of placing a steak dinner into a trash heap. It is likelier that this will make the meal inedible than make the trash heap delicious.
Another major area of concern is the impact Informa’s deal may have on intellectual property rights. Will anyone with a generative AI subscription be able to rip off scholarly work without attribution? To its credit, Informa insists in the announcement that “the agreement protects intellectual property rights, including limits on verbatim text extracts and alignment on the importance of detailed citation references.” But two questions immediately come to mind: Can publishers like Informa actually prevent plagiarism from generative AI, and how serious are they about doing so? As to the first question, Informa has not released further details on how they will live up to their commitment to protect the content provided to generative AI systems. While fears of the widespread proliferation of plagiarism among students using generative AI are likely overstated, we know these systems can and do use work without attribution.
While there are therefore serious questions about the ability of publishers and their generative AI tech company partners to offer meaningful protections of intellectual property rights, the woefully inadequate description of plagiarism contained within the announcement leads to questions about the sincerity of Informa’s commitment. The precise wording of the announcement, centered on “verbatim text extracts,” is troubling. Most scholars would define plagiarism as any uncredited use of someone else’s ideas. While this certainly includes blatant abuses such as word-for-word copying, it also includes summarizing and paraphrasing—indeed, any form of unattributed use—which are not mentioned in Informa’s statement.
And the emphasis in the statement on “alignment on the importance of detailed citation references” reveals Informa’s dependence on third-party tech companies to meet high standards of academic citation practices. With the proliferation of LLMs, there are bound to be exceptions, but so far there is little reason for optimism that for-profit generative AI companies on the whole share a commitment to the norms of scholarly attribution. Instead, these companies often pay lip service to ethical principles while, in practice, they are engaged in an exceedingly lucrative process of extracting information with little transparency.
For scholars thinking about signing book contracts in future, there are also unresolved questions about what this means for their earnings potential and career prospects. Traditional book contracts are based on numbers of copies sold. Publishers may sell scholarly books in electronic formats, which are licensed for periods of time by public or academic libraries. Even in these new forms of dissemination, the number of times a book is accessed can be easily tracked. Moreover, all of these media reproduce works in their entirety.
Training an LLM on scholarly research is categorically different. The resultant algorithm could break down academic work into constituent parts, combine it with other information that the LLM has trained on and instantly reconfigure the work as it sees fit. It is not clear how the use of any work could be tracked individually since LLMs train on information on a gargantuan scale. Informa insists that academics will be paid royalties as part of this deal, but given the radical difference between training an LLM on a scholarly work and traditional methods of publication, how this will be done, and on what terms, is still up in the air.
Except for a few superstars, most academics already expect little monetary compensation for the labor of writing, so an even more pressing question is what this means for scholarly advancement. On the one hand, the idea that anyone with a generative AI subscription could now have access to a scholar’s work could significantly increase the potential impact of scholarship. But will the outputs of generative AI contain the same level of rigor, nuance and complexity that are the hallmark of academic writing? And, given the potential problems of attribution noted above, will this impact be visible?
Scholars at research institutions rely on numbers of citations, downloads or other metrics to measure the impact of their scholarship. Since AI systems train on vast amounts of data, in which any given book or article would be a mere drop in the bucket, it is not yet clear how it would be possible to provide a reasonable measure of scholarly impact.
When I signed my last book contract, I was on the academic job market and needed to demonstrate the strength of my scholarly record to search committees. As a result, like so many other scholars looking for jobs, experiencing precarity of one stripe or another, desperate to get tenure, or move up the scholarly ranks, I had precious little leverage in the book negotiation process. I knew that my publisher was interested above all in the profits they could earn from my scholarship, but—naively, it now seems—I assumed that in other respects our interests aligned. To maximize their profits, I presumed, they were incentivized both to promote the exposure of my work and to protect my intellectual property.
Informa’s deal to feed their massive corpus of scholarship to a generative AI system, a deal which they made without consulting authors, calls these assumptions into question. If authors can expect neither profit nor exposure from their scholarship, academia’s mantra in the new arena of AI and academic publishing may well be to publish and perish.