You have /5 articles left.
Sign up for a free account or log in.
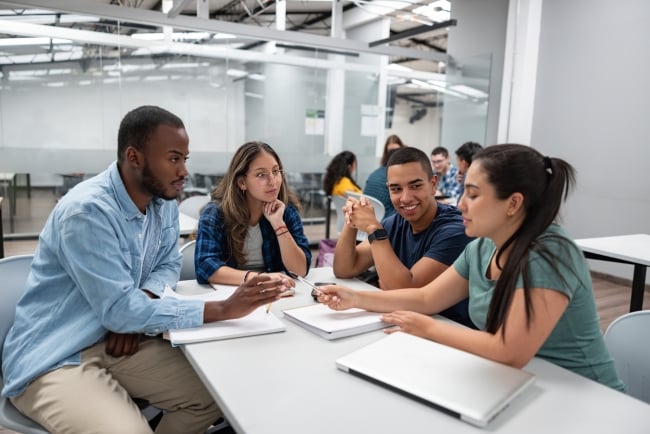
Predictive analysis models commonly used in higher education may have a bias against learners from some racial and ethnic minorities based on limited data sets and other systemic inequities.
andresr/E+/Getty Images
Predictive models are used across the student life cycle in higher education, to gauge yield in admissions as well as retention and graduation initiatives, as campus leaders look to understand what factors improve student success.
Early alert systems can benefit learners by helping guide them back on track, but not all flags are indicative of students’ trajectories and may instead be raising false alarms.
A research article titled “Inside the Black Box: Detecting and Mitigating Algorithmic Bias Across Racialized Groups in College Student-Success Prediction,” published in AERA Open, the American Educational Research Association’s journal, sought to understand if predictive models perpetuate social disparities, such as racism, sexism and classism.
Researchers found some models predict outcomes for Black and Hispanic students incorrectly—largely for the worst—as well as overestimate the potential success of white and Asian students. Measures that modify algorithms to incorporate fairness reduced disparities in predictions, but none of the techniques eliminated disparities in either prediction outcomes or accuracy.
The background: A 2018 NASPA survey of 970 professionals at colleges and universities found 89 percent of respondents had made some investment in predictive analytics. Predictive models are computational tools that use a set of student attributes and their outcomes to find underlying patterns and associations. These attributes could be student achievements, like high school GPA, or demographic factors.
More recently, equity in predictive analytics has become a concern in higher education. Due to larger societal injustices, students with socially relevant features (race, gender or income, for example) may receive less favorable outcomes from the predictive model. Previous research has found most algorithms in student success have higher false negative rates (predicting failure for students who graduate with a bachelor’s) for racially minoritized students.
Researchers set out to understand algorithmic bias present in predictive analytics by evaluating how predictions vary by racial and ethnic groups, how the models’ accuracy varies, and if common techniques to mitigate bias are effective.
Accuracy was a key point in the research because it could point to errors in data for racially minoritized students, missing variables that predict success for those students or if successful students are underrepresented in historical data used to train the model.
Methodology: The study pulled from the U.S. Department of Education’s National Center for Education Statistics Educational Longitudinal Study of 2002 from the past 10 years, including over 15,240 students. Student success was marked as a student’s attainment of at least a bachelor’s degree within eight years after expected high school graduation.
Researchers employed 27 predictive variables, including demographic characteristics, socioeconomic traits, grades, college preparation and K-12–level contextual variables. The study also implemented four statistical techniques to mitigate bias in preprocessing and in-processing.
Equal opportunity would be shown if each group had similar false negative rates between groups.
The results: There were four results a student could receive from the algorithm:
- A true positive (predicted success for a student who succeeds)
- A true negative (predicted failure for a student who does not succeed)
- A false positive (predicted success for a student who does not succeed)
- A false negative (predicting failure for a student who succeeds)
The models incorrectly predicted failure for Black and Hispanic students 19 and 21 percent of the time, respectively. In comparison, white and Asian students were given false negatives 12 percent and 6 percent of the time.
Additionally, the models were inaccurate in their predictions of whether a student would complete their degree. Among students who did not complete, white students and Asian students were more likely to be predicted successful (65 percent and 73 percent, respectively), compared to their Hispanic and Black peers (28 percent and 33 percent, respectively).
The model also had a greater variation of unfairness for Hispanic and Black students and those from two or more races due to the population bias across the data set.
So what? “The practical implications of these findings are significant, but depend on the use of predictive outcomes,” researchers wrote. For example, in admissions decisions, predictive models may result in unfair rejections for students from racially minoritized backgrounds if they receive lower predicted likelihoods of success. Advisers may be less likely to recommend courses or majors to students if the academic path is perceived as challenging or students are predicted to fail based on the statistical model.
False predictions may also result in greater investment in resources for disadvantaged students who may otherwise succeed, so practitioners should be careful not to perpetuate deficit narratives of minoritized students or treat them as though they have a lower probability of success, authors caution.
“Awareness of potential algorithmic bias, including its direction and the groups targeted by the bias, can help users contextualize predictions for individual students and make more informed decisions,” according to the study.
Researchers did find that there can be ways to mitigate algorithmic bias for practitioners, but this does not close equity gaps entirely, so they advocate for additional research in how to reduce algorithmic bias across racialized groups.
Get more content like this directly to your inbox every weekday morning. Subscribe here.